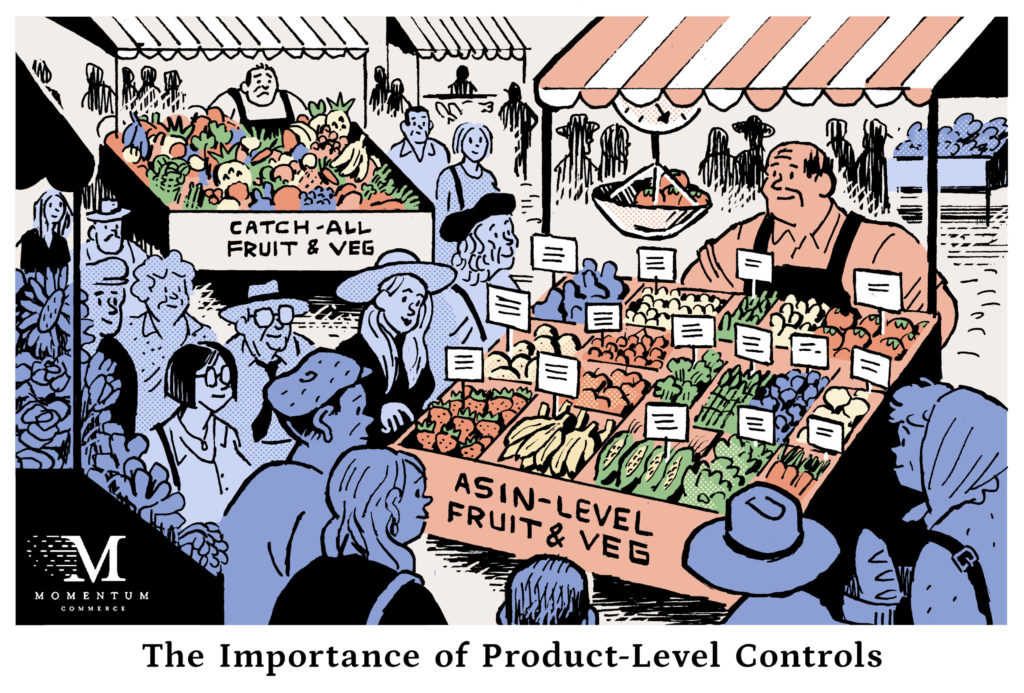
The Importance of Product-Level Control in Amazon Advertising
Your customers don’t buy keywords. They don’t buy ad placements. They don’t buy ad types. And they definitely don’t buy your SB headline copy. They buy your products. Understanding how your products are performing, and what is driving that product performance is the most important and fundamental responsibility of any marketer on Amazon.
The problem all started when Amazon decided that you should not be able to produce a table that is structured in the following way with reports available in the Ad Console:

You can only ever view one of the Dimensions (Keyword and ASIN) at a time.
Even if you had this data, there is not an effective approach to modifying bids at the Keyword level based on Keyword to Product performance data. You must isolate your bid changes to the Keyword to Product pair, and the only way this is possible is through sound Product-level Campaign and Ad Group architecture, specifically one product per Ad Group.
To invent an imaginary example to show exactly where the gaps are – using the Purchased Product Report you would be able to produce a table like the one below for a given Keyword, which in this case has delivered advertising performance for 5 distinct ASINs:
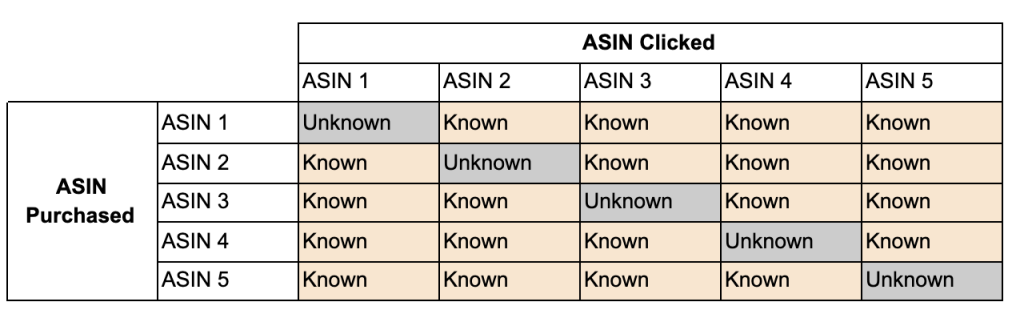
The values in Orange are situations where you can know definitively the sales resulting from a click on a product. The values in grey, unfortunately, are unknowable through the reports Amazon makes available. In other words, you can never know with absolute certainty the sales for a specific ASIN that resulted from a click on that same ASIN and what the Keyword was that drove the click.
Now, it looks like Amazon has tried to make this data available – although unsuccessfully. In the ASIN Report in their Advertising API there is the concept of `ASIN` and `otherASIN` as well as `AttributedUnitsOrdered` and `AttributedUnitsOrderedOtherSKU` and most importantly `KW ID` and `KW Text`. That said, from what I can discern in my (admittedly naive) testing of this data, all values in the `AttributedUnitsOrdered` column are reported as 0, while the OtherSKU metrics are the only fields to have real values. So it’s essentially useless.
But let’s just take a quick trip to Perfect World – and imagine we knew the Sales and Spend values for each KW at the Product Level. You still need to control bids at the KW level – they are not set at the Product level – and this reminds us how important it is to structure your account at the product level.
Still in Perfect World – below is an example of what I would argue is a poorly structured Ad Group. It contains three products.
Ad Group 1
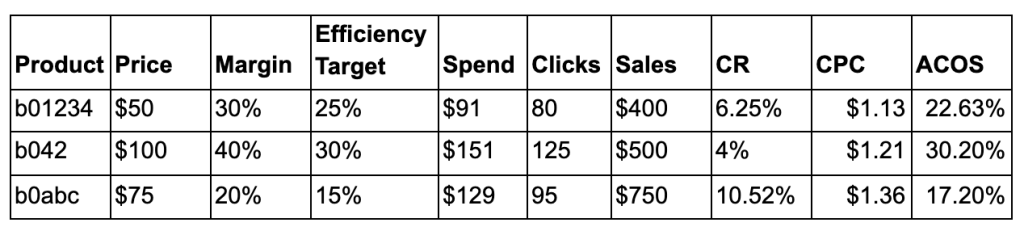
We can calculate the resulting Revenue Per Click (RPC) and Value Per Click (VPC) to help us determine what the optimal bids might be.
Formula for RPC = Sales / Clicks
Formula for VPC = RPC * Efficiency Target
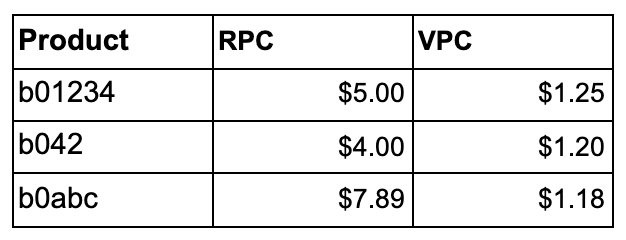
Unfortunately, this tells us what the optimal bid would be at the product level, not the Keyword level where we actually have to make the bid adjustment.
Using our Perfect World Keyword-ASIN paired data we will have the below table available. For the purposes of this exercise, we are going to assume that we pay exactly what we bid, but the conclusions will remain true even in a more realistic second-price setting.
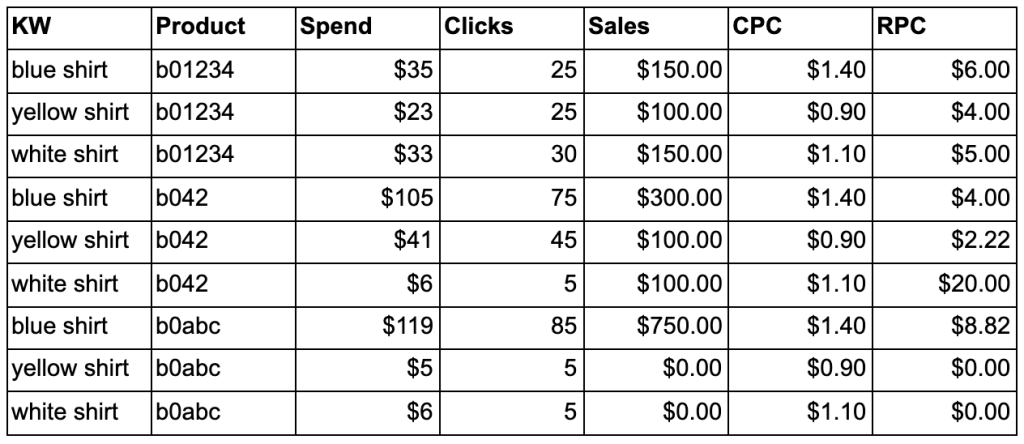
We can then take this data and calculate new optimal bids (VPC) at the Keyword AND Product level, and answer the key question: ‘what is the right bid for a particular KW-Product Pair?’
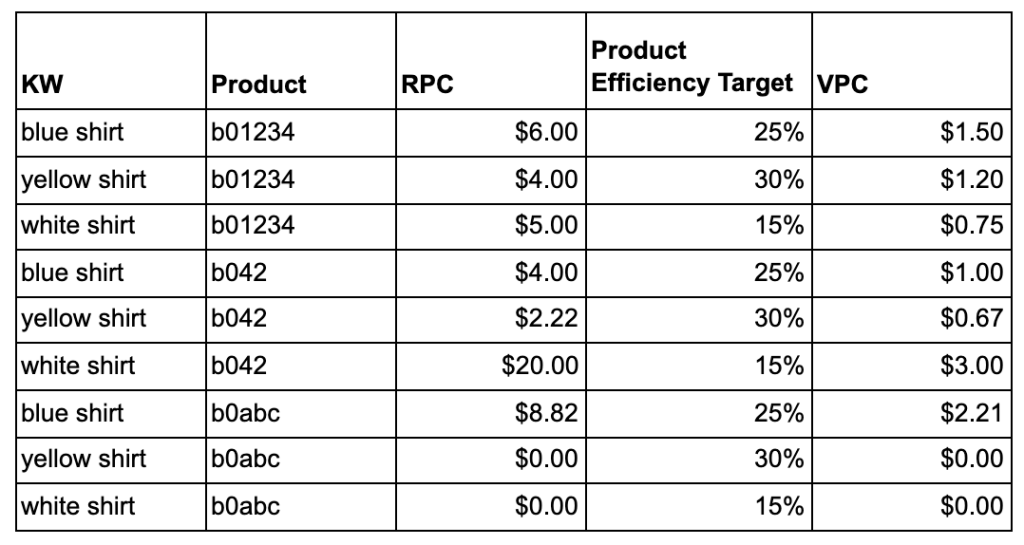
Again, we are still faced with the challenge that we cannot update Keyword level bids conditionally per Product when each of these products exists in the same Ad Group. The KW ‘Blue Shirt’ has 3 different VPCs ($1.50, $1.00, and $2.21) depending on the Product that was advertised.
Let’s try to brute force it anyway – using the average values to calculate distinctly Keyword level bid changes. As an example, we could take a weighted proportion of clicks per product per Keyword, and then distribute the optimal bid values at the Keyword level. The math would look like this:
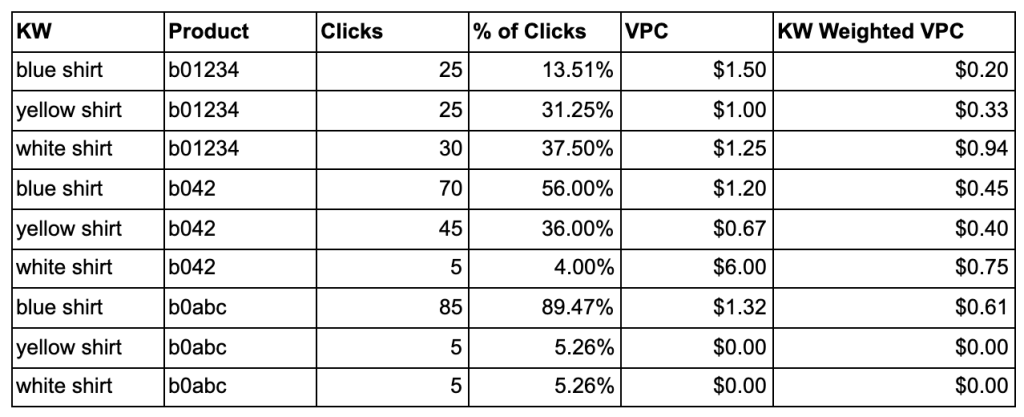
Then by summing the resulting Keyword Weighted VPC values, this approach would result in the following changes:
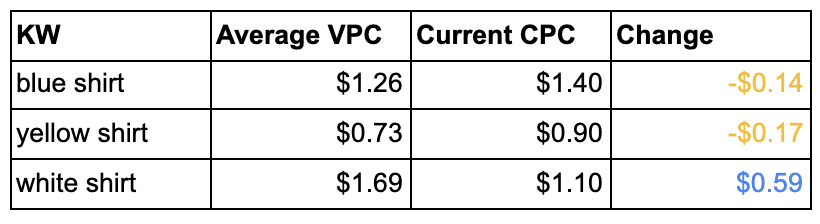
In this scenario we are decreasing the bid value and CPC for the ‘Blue Shirt’ keyword for all products, even though it is performing better than the optimal bid level for the b01234 item. Conversely, we would massively increase the bid on the ‘white shirt’ Keyword by nearly $0.60 while only the b042 item is performing well for this Keyword with a very low click count. Ultimately, applying this bid methodology to these 3 KWs will yield ever changing Product level VPCs as click counts and CRs change with the new bids in place and the proportion of clicks per product will shift. In other words, our bid changes will have causally modified what the bid should have been. More simply, these bid changes only improve the bid accuracy for some products at the expense of accuracy for others.
Taking a simpler approach of only using an unweighted average of the optimal VPC does not improve the bid effectiveness, and actually compounds the ‘white shirt’ problem to an even larger bid increase.
There may be other ways you can combine averaged values to calculate more accurate bid changes for multi-product to Keyword situations. I was not able to find one that worked more effectively than simply splitting the Keywords and Product Pairs into their own Ad Groups to allow you to bid discretely on each pair.
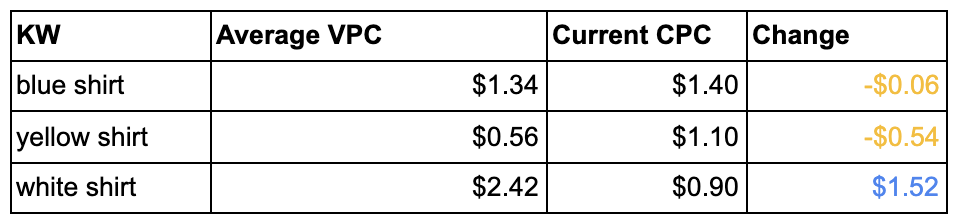
Using current methods, there is not an effective approach to modifying bids at the Keyword level based on Keyword to Product performance data. You must isolate your bid changes to the KW to Product pair, and the only way this is possible is through sound Product level Campaign and Ad Group architecture. The ideal structure is 1 parent product per Ad Group. I will sometimes allow multiple parent ASINs to be grouped together in an Ad Group in the case of very high product count catalogs that we typically see in the Softlines vertical – but the goal must be to cluster products based on expected CR similarity for the set of KWs that will belong to the ad group.